What Is Dfs Algorithm in Data Structures
DFS stands for Depth First Search. Generally, it is a repetitive or decidable type of algorithm which is basically used in identifying all the vertices or nodes of a graph or tree data structure. It begins its hunt from the initial node in the graph G and hunts deeper and deeper until it reaches its goal which is also known as the goal node or the node that has no children. Due to its repetitive or to be more precise recursive behavior, the stack data structure is used in order to implement the DFS algorithm.
The stack data structure is generally used for this kind of traversal. The stack data structure works on the LIFO principle. LIFO stands for ‘Last In First Out’. In this type of algorithm, we can generally begin from any given node or anywhere unless and until the root node is mentioned in the problem. We can consider any node as the root node.
Here are the various steps following which we can apply DFS algorithm to solve any problem:-
- The very first step is to construct a stack with total number of vertices in the graph.
- Then, we can begin by choosing any particular vertex as the initial node and push that vertex into the stack.
- Now, we can move ahead and push any non-explored vertex to the top of the stack.
- Next, we just have to repeat the steps 2 and 3 till we are left with no such vertex that we have not explored yet.
- After a while, when there is no such vertex left, then you can move back to the top and pop a vertex from the stack.
- Keep repeating steps 2,3 and 4 until the stack is completely empty.
APPLICATIONS OF DEPTH FIRST SEARCH
In this section, we are going to discuss the applications and uses of the depth first search algorithm in our day to day lives. The following are its applications:-
- It is generally used to find the track or route among the two vertices.
- They are also used in indentifying cycles in the graph.
- They are also used in solving one solution riddles.
- They are used in implementing geological sorting.
- It is also used for its application in finding out whether a graph is bipartite or not.
ADVANTAGES OF DFS
- DFS is known for its very less consumption of memory space. This is generally due to the fact that the nodes which are present on the current path, only those nodes are stored.
- It also has the capability of reaching the goal node in a very less time if it traverses on the right path.
- It finds a solution without having to traverse much, say in the very first go.
- It stops finding solution when it finds at least one of them.
DISADVANTAGES OF DFS
- It seems to be a possibility that there might exist many states and they might reappear as there will be no guarantee of finding the goal node.
- It is also possible that these states might enter into an infinite loop.
IMPLEMENTATION OF DFS
Suppose we take an example in order to explain the DFS algorithm.
Suppose we take P as the root or initial node and Z as the goal node, then we have to follow the following steps:-
Step 1: Start by pushing the initial node or H onto the stack.
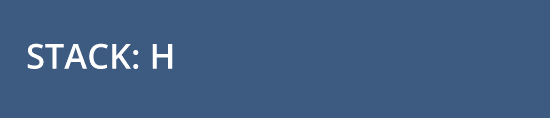
Step 2: Now, we can pop the element from stack that is H and all its adjacent nodes.
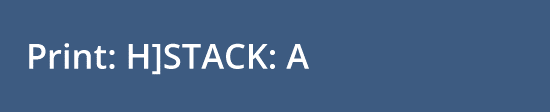
Step 3: Next, we can pop the element from stack that is A and all its adjacent nodes.
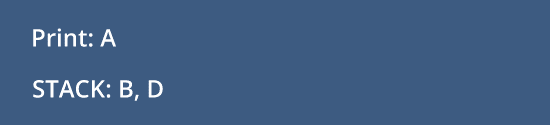
Step 4: Now, we will POP the element D and print it and push all the adjacent nodes of D into the stack.
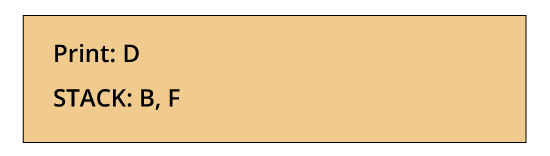
Step 5: Next, we will POP the element F and print it and push all the adjacent nodes of F into the stack.
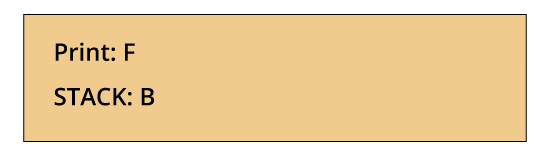
Step 6: Now, we will POP the element B and print it and push all the adjacent nodes of B into the stack.
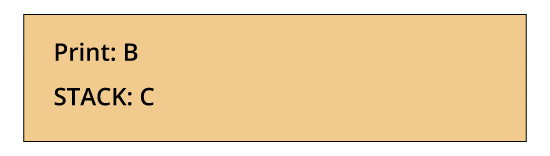
Step 7: Now, we will POP the element C and print it and push all the adjacent nodes of C into the stack.
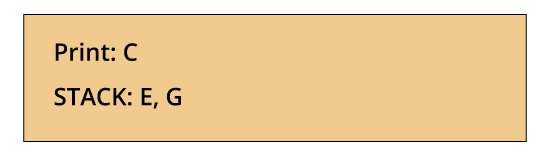
Step 8: Now, we will POP the element G and print it and push all the adjacent nodes of G into the stack.
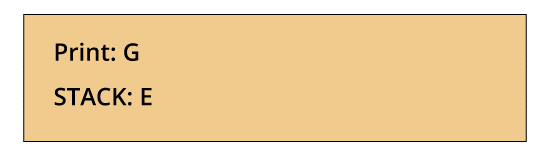
Step 9: Now, we will POP the element E and print it and push all the adjacent nodes of E into the stack.
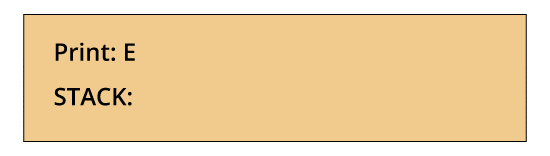
PSEUDOCODE FOR DFS
DFS(G,x) (x is the vertex from where the search will begin)
STACK Z :={}; (begin with an empty stack)
For each vertex y, set visited[y] := false;
push Z, x;
while (S is not empty) do
y:= pop Z;
if (not visited[y]) then
visited[y] := true;
for each unvisited neighbour w of yy
push Z, w;
end if
end while
END DFS()
Output:
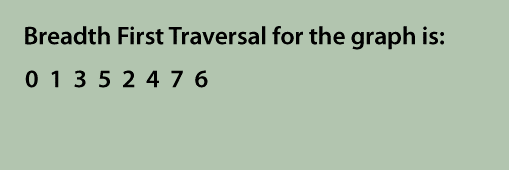
COMPLEXITY OF DFS
We know that when we are talking about the complexity of DFS, we are mainly talking about the time complexity. The time complexity of this algorithm when the entire tree is explored or traversed is considered to be O(V) and we know that V stands for the total number of nodes present in that file.
Each and every node keeps a check on the list of all its neighbouring nodes and edges. Let us suppose that there are V number of nodes and E number of edges present. For every single node we explore the all the adjacent by exploring its list only a single linear time. If we talk about a directed graph, the sum of all the sizes of list that contains all the nodes is E. So, in this case the complexity will be O(V) + O(E) = O(V+E). Whereas for an undirected graph, each and every edge presents itself atleast twice. Either at the end of the list where the complexity will be O(V)+ O(2E) ~ O(V+E). if the graph is presented as a matrix then, each row is considered to be a node in the graph and that particular row is known for storing diverse information especially about the edges emerging from the node and in this case the complexity tends to be O(V*V)= O(V2).